Learning About Machine Learning: A Transformative Business Tool
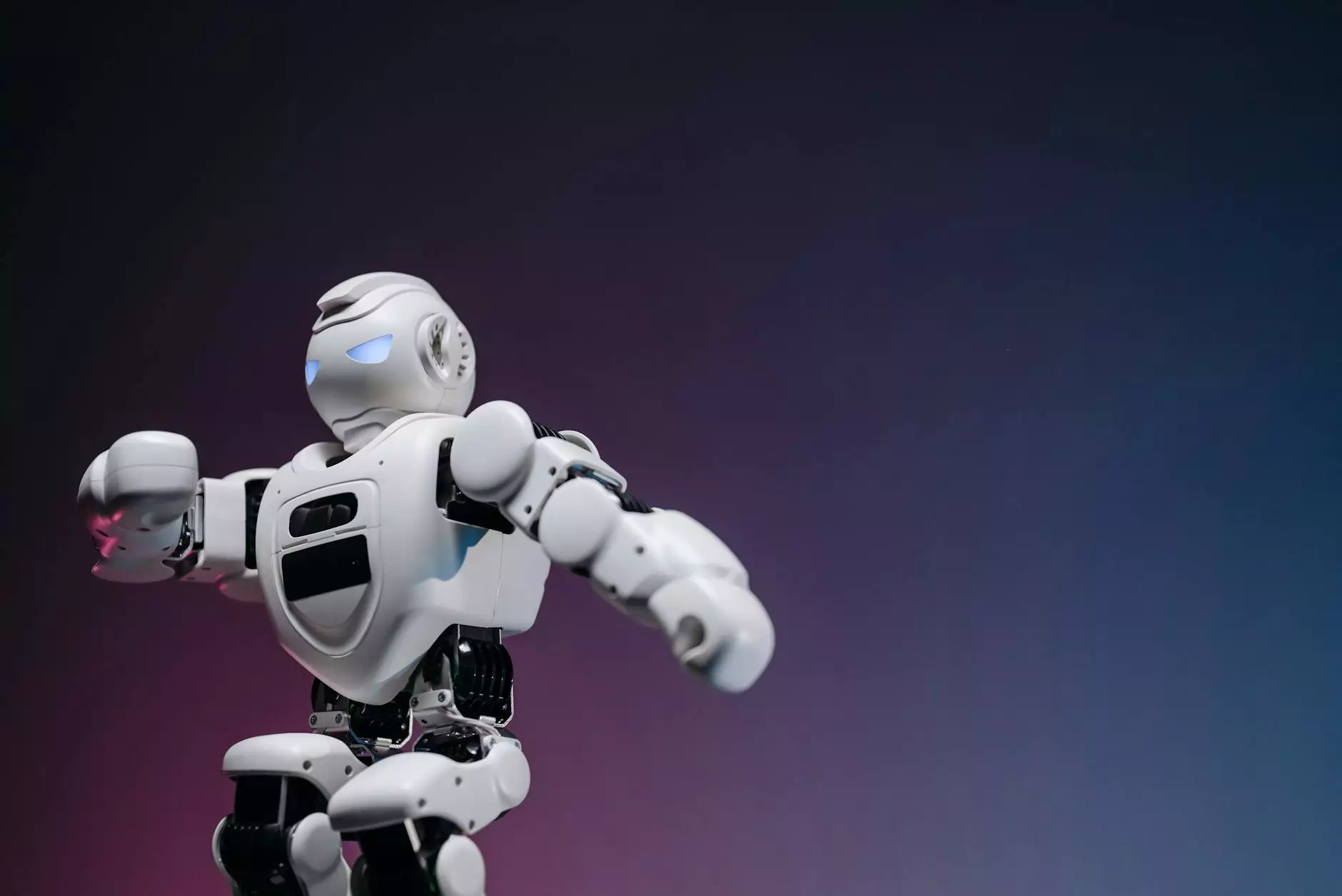
In today's rapidly evolving digital landscape, the implementation of technology, notably machine learning, has become a cornerstone for business advancement. The concept of learning about machine learning is not just a trend; it's a strategic approach that can redefine how businesses operate.
The Significance of Machine Learning in Modern Business
Machine learning, a subset of artificial intelligence (AI), enables systems to learn and adapt through data. It has far-reaching implications across various sectors, making it essential for businesses aiming to stay competitive. Here’s why learning about machine learning is crucial:
- Data-Driven Decision Making: Machine learning provides businesses with the ability to analyze vast datasets, unveiling patterns and trends that can drive strategic decision-making.
- Enhanced Customer Experiences: Through predictive analytics, companies can better understand customer behaviors, leading to more personalized experiences and improved satisfaction.
- Operational Efficiency: Automation of routine tasks and optimization of operations are made possible through machine learning, resulting in reduced costs and increased productivity.
- Innovation and Competitive Edge: Businesses that leverage machine learning can innovate faster and outperform competitors by adapting swiftly to market changes.
Understanding Machine Learning: Key Concepts
Before diving into how businesses can implement machine learning, it’s crucial to understand some foundational concepts:
1. What is Machine Learning?
Machine learning is a field of computer science that focuses on building systems that learn from data, identifying patterns, and making decisions with minimal human intervention.
2. Types of Machine Learning
- Supervised Learning: Involves training a model on a labeled dataset, where the outcome is known.
- Unsupervised Learning: Involves training a model without labeled outcomes, focusing on finding hidden patterns or intrinsic structures in input data.
- Reinforcement Learning: This type is based on agents that take actions in an environment to maximize some notion of cumulative reward.
3. Key Algorithms in Machine Learning
Understanding common algorithms can significantly enhance your ability to apply machine learning in a business context. Some important algorithms include:
- Linear Regression: Used for predicting continuous outcomes.
- Decision Trees: Provide a visual representation of decisions and their possible consequences.
- Neural Networks: Particularly effective for complex problems like image and speech recognition.
- Support Vector Machines (SVM): Effective in high-dimensional spaces for classification tasks.
Implementing Machine Learning in Your Business Strategy
To harness the power of machine learning, businesses must take a strategic approach. Here are the essential steps for implementing machine learning:
1. Identify Business Problems
The first step is to clearly define the business problems that can benefit from machine learning solutions. Possible areas include:
- Fraud detection and prevention
- Predictive maintenance
- Customer segmentation
- Sales forecasting
2. Data Collection and Preparation
Machine learning is heavily dependent on data. Collecting and preparing the right data in the right format is crucial:
- Ensure data quality to avoid errors in outcomes.
- Use diverse datasets to improve model accuracy.
- Implement data preprocessing techniques like normalization, encoding categorical variables, and dealing with missing values.
3. Model Selection
Based on the problem defined and the nature of the data, select an appropriate machine learning model. Experiment with different models to:
- Evaluate their performance through cross-validation.
- Consider the trade-offs between model complexity and interpretability.
4. Training and Testing the Model
Once a model is selected, it must be trained with the dataset and tested to evaluate performance. Techniques include:
- Splitting the dataset into training and testing sets.
- Utilizing metrics like accuracy, precision, recall, and F1 score to measure effectiveness.
5. Deployment and Monitoring
Deploy the model into production and monitor its performance. Continuous monitoring is essential to:
- Identify model drift as new data comes in.
- Ensure the model remains effective and relevant over time.
Real-World Applications of Machine Learning in Business
Several companies have successfully integrated machine learning into their operations. Here are some notable examples:
1. Amazon
Amazon utilizes machine learning for various applications, including:
- Product Recommendation: The recommendation engine analyzes user behavior to suggest products, thereby increasing sales.
- Inventory Management: Predictive analytics ensures optimal stock levels based on demand forecasting.
2. Netflix
Netflix employs machine learning to enhance user experience through:
- Content Personalization: Personalized recommendations based on viewing history.
- Churn Prediction: Identifying users likely to cancel subscriptions and implementing retention strategies.
3. Uber
Uber leverages machine learning for:
- Dynamic Pricing: Adjusting ride prices based on demand and supply through predictive modeling.
- Route Optimization: Machine learning algorithms analyze traffic patterns to provide the best travel routes.
Challenges and Considerations in Machine Learning
While the benefits are substantial, businesses must also navigate several challenges when exploring machine learning:
1. Data Privacy and Security
With increasing regulations regarding data privacy, businesses must ensure compliance when collecting and using customer data.
2. Talent Shortage
A skilled workforce is essential for successful machine learning implementation. Companies may face challenges in recruiting data scientists and ML experts.
3. Integration with Existing Systems
Integrating machine learning models with legacy systems can be complex and requires careful planning and execution.
Future Trends in Machine Learning
The future of machine learning in business holds several exciting trends:
- Automated Machine Learning (AutoML): Reducing the barrier to entry for non-experts and facilitating faster model development.
- Explainable AI: Focusing on transparency and interpretability of AI decisions to increase trust.
- Federated Learning: Allowing for model training on decentralized data sources to enhance privacy and security.
Conclusion
In conclusion, learning about machine learning is an imperative for any business seeking to thrive in the modern economy. By understanding its concepts, implementing strategic approaches, and staying abreast of emerging trends, companies can unlock unprecedented opportunities for growth and efficiency. The potential of machine learning will only continue to expand, making now the ideal time to invest in this transformative technology.
For more insights and consultation on effectively integrating machine learning into your business strategy, visit machinelearningconsulting.net.