The Transformative Impact of Image Annotation Tools for Machine Learning
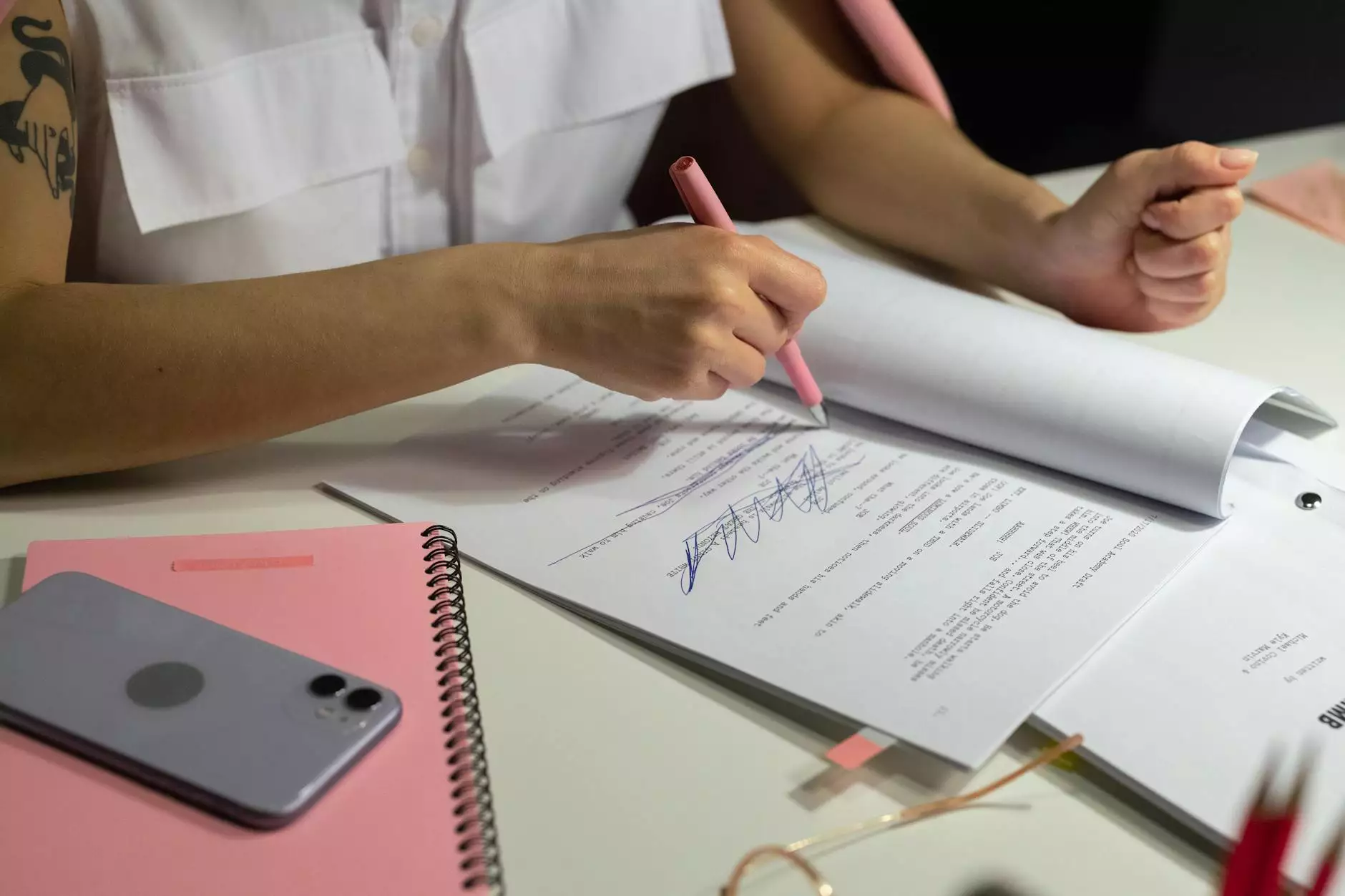
In the evolving landscape of machine learning and artificial intelligence, the significance of accurate data representation cannot be overstated. As organizations strive to leverage machine learning to its fullest potential, the demand for efficient, precise data labeling has surged. This brings us to the critical role of image annotation tools for machine learning. These tools serve as the backbone for training algorithms through well-defined datasets, ultimately contributing to better performance and accuracy in predictive models.
Understanding Image Annotation and Its Relevance
Image annotation is the process of labeling images with key details that help in teaching machines to recognize patterns, objects, and activities. This is particularly important in numerous fields such as healthcare, automotive, and security. The effectiveness of the machine learning model heavily relies on quality annotations, which will directly influence its learning curve and prediction accuracy.
What is an Image Annotation Tool?
An image annotation tool is a software application designed to facilitate the process of adding labels, metadata, and other relevant information to images. These tools make it possible to accurately mark various elements within a visual, including:
- Elements Identification: Marking specific objects or features.
- Bounding Boxes: Drawing boxes around identified objects.
- Segmentation: Dividing images into segments for more precise labeling.
- Landmark Points: Highlighting critical points like facial features in images.
- Classification Tags: Categorizing images based on distinctive features.
The Importance of Accurate Image Annotations
The reliability of a machine learning model hinges largely on the accuracy and quality of the image annotations. Here are key reasons why precise annotations are imperative:
- Enhanced Model Performance: High-quality annotations lead to better training outcomes, resulting in models that understand and interpret data more effectively.
- Reduced Errors: Properly annotated datasets minimize misclassification, which can skew results and lead to erroneous conclusions.
- Faster Development Cycles: Efficient image annotation processes allow for quicker iteration, driving rapid advancements in product development.
- Scalability: A robust annotation framework allows organizations to scale their operations effectively, adapting to increased data needs without compromising quality.
Key Features of Image Annotation Tools
When selecting an image annotation tool for machine learning needs, there are several features you should consider to ensure you choose the right platform:
1. User-Friendly Interface
A tool that is intuitive and easy to use will save time and reduce training costs for new users. An accessible interface encourages wider adoption across teams.
2. Supports Various Annotation Types
Your chosen tool should facilitate diverse annotation types, including semantic segmentation, keypoint identification, and bounding box creation, to accommodate various machine learning applications.
3. Integration Capabilities
The ability for the annotation tool to integrate with other systems and platforms is critical. This ensures a smooth workflow and maintains the consistency of data across platforms.
4. Quality Assurance Features
Built-in quality control mechanisms help verify the accuracy of annotations, ensuring that you maintain high standards for your training datasets.
5. Collaboration Support
For teams working on large-scale projects, collaborative features allow multiple users to work on parts of the same dataset, enhancing productivity and fostering team work.
Popular Image Annotation Tools: A Comparative Overview
Several image annotation tools dominate the market, each offering unique features that cater to various user needs. Below are a few of the most widely used tools in the industry:
1. Labelbox
Labelbox is a powerful platform for image annotation that offers a suite of features including collaboration tools and integration with machine learning workflows.
2. VGG Image Annotator (VIA)
The VGG Image Annotator is a free, open-source tool, ideal for users who want a lightweight application for basic annotation tasks.
3. RectLabel
RectLabel is a popular image annotation tool for Mac that supports video annotation as well, making it a versatile choice for multimedia projects.
4. Supervisely
Designed for teams, Supervisely provides a comprehensive platform that supports not only image annotation but also video and 3D point cloud annotations.
Industries Leveraging Image Annotation Tools for Machine Learning
The application of image annotation spans various industries, illustrating its versatility and importance:
1. Healthcare
In healthcare, image annotation aids in the detail-oriented fields of radiology and medical imaging. Annotated images help medical professionals train algorithms to detect abnormalities, thus improving diagnostic accuracy.
2. Automotive
For the automotive industry, especially in autonomous vehicles, image annotation is critical. Accurate labeling of road signs, vehicles, and pedestrians ensure the safety and efficiency of AI-driven cars.
3. Retail and E-Commerce
In retail, image annotation improves inventory management and product recognition systems. Annotated images help AI understand products better, which enhances online shopping experiences.
4. Security and Surveillance
Security systems utilize image annotation for facial recognition technology. By training models on accurately annotated images, these systems can improve their ability to identify individuals in varying conditions.
The Future of Image Annotation Tools in Machine Learning
As machine learning continues to advance, the future of image annotation tools looks promising. Innovations such as deep learning and automation are likely to redefine how businesses approach data labeling.
Integration of AI in Annotation Tools
Emerging trends show that future annotation tools will likely incorporate AI for automated annotations, further enhancing efficiency and reducing human error. Such integration could revolutionize the data preparation phase, allowing data scientists to focus more on model development.
Cloud-Based Annotation Platforms
The rise of cloud technology will facilitate greater collaboration among distributed teams, streamlining the annotation process and providing instant access to resources from anywhere in the world.
Adaptation to Industry-Specific Needs
As industries become more specialized, annotation tools are expected to evolve, offering tailored features catering to specific sector requirements, whether in healthcare, automotive, or retail.
Conclusion: The Critical Role of Image Annotation Tools in Machine Learning
In conclusion, image annotation tools for machine learning are indispensable in today’s data-driven landscape. They empower organizations to harness the full potential of machine learning by enabling effective training of models through high-quality labeled datasets. As technology continues to evolve, the importance of accurate annotations will only grow, driving innovations that enhance efficiency and productivity across industries.
Whether you are in healthcare, automotive, retail, or any other sector leveraging AI, investing in an effective image annotation tool is crucial for achieving success in your machine learning endeavors.