Revolutionizing Data Annotation with Image Segmentation Labeling Tools
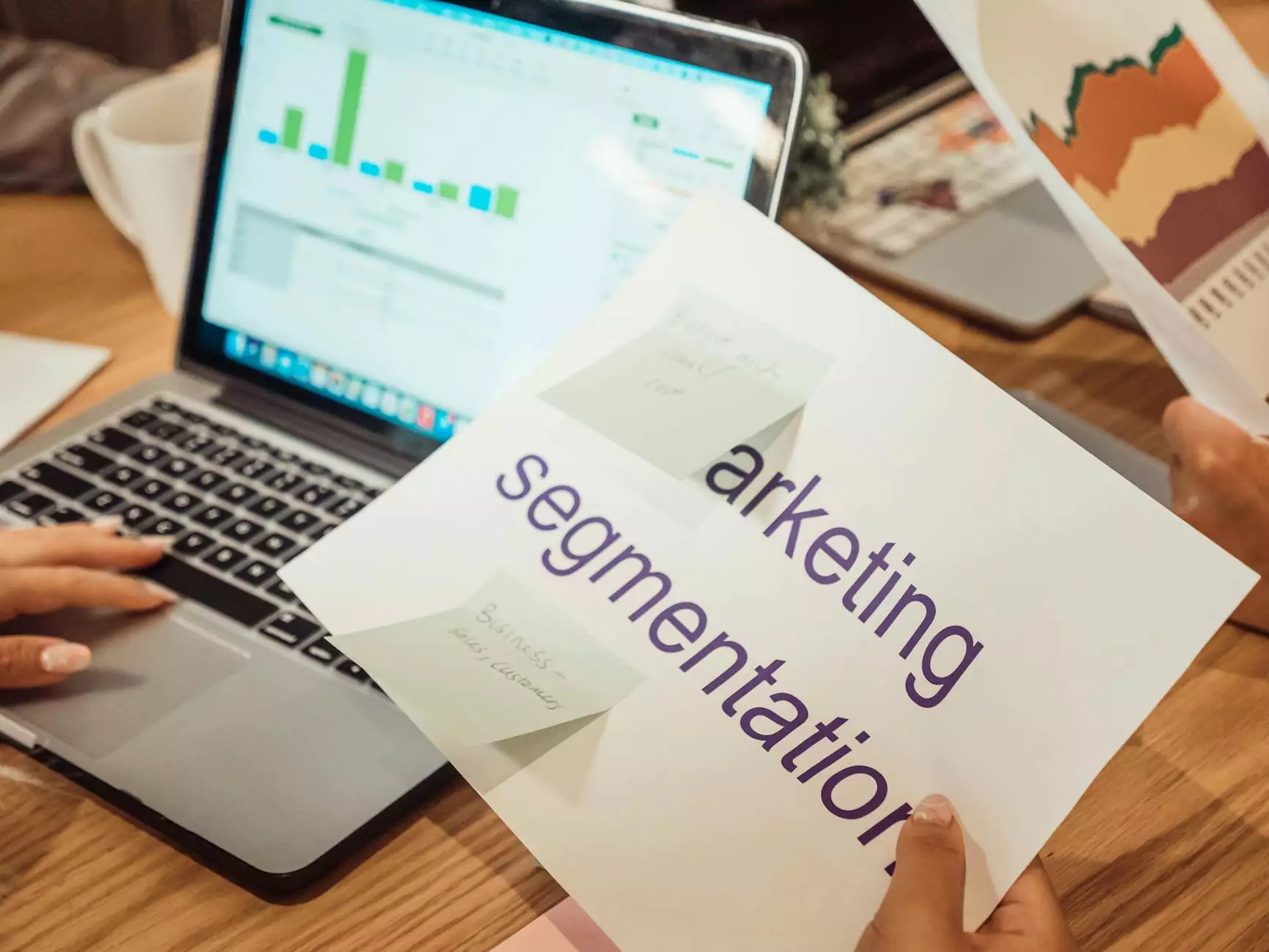
The rise of artificial intelligence and machine learning has significantly impacted how businesses operate across various sectors. One of the most crucial aspects of training AI models is data annotation, which involves labeling data accurately. This is where an image segmentation labeling tool plays a pivotal role. In this article, we will delve deep into what these tools are, their benefits, and how they can elevate your business operations, especially in the domain of data annotation.
Understanding Image Segmentation
Image segmentation is the process of partitioning an image into multiple segments to simplify its representation and analyze it effectively. This allows for easier identification of objects and boundaries within images. When referring to image segmentation in the context of AI, it generally falls under two categories:
- Semantic Segmentation: This approach classifies each pixel in an image as belonging to a certain class, effectively labeling regions of an image based on object categories.
- Instance Segmentation: This is a more advanced method that not only classifies each pixel but also differentiates between separate objects of the same class, allowing for precise object detection.
The Importance of Data Annotation
Before we dive into the specifics of image segmentation labeling tools, it’s essential to understand why data annotation is crucial. Data annotation is the backbone of any AI system, providing the necessary structured data that algorithms require to learn and make predictions. The quality of AI models directly correlates with the quality of the labeled data they are trained on. Here are some reasons why data annotation matters:
- Accuracy: High-quality labeled data significantly improves the accuracy of machine learning models.
- Efficiency: Proper data annotation saves time and resources in the long run by reducing the need for extensive retraining.
- Scalability: Efficiently labeled data allows for scalable solutions that can adapt and learn from new data inputs.
What is an Image Segmentation Labeling Tool?
An image segmentation labeling tool is specialized software designed to assist in the annotation process of images through segmentation techniques. These tools vary in complexity and capability, allowing users ranging from entry-level data annotators to seasoned data scientists to effectively label images with high accuracy. They typically come equipped with features that enhance user experience, speed up the annotation process, and ensure data integrity.
Key Features of a Quality Image Segmentation Labeling Tool
When considering an image segmentation labeling tool for your data annotation needs, certain features can greatly enhance your workflow and output quality. Here are some essential features to look for:
- User-Friendly Interface: A clean and intuitive interface allows users to navigate the tool without steep learning curves.
- Automated Annotation: Machine learning-driven features that can suggest annotations based on existing data can drastically improve efficiency.
- Collaboration Tools: Multi-user capabilities enable teams to work together seamlessly, ensuring consistency and collaborative efforts in data labeling.
- Quality Assurance Mechanisms: Built-in review and validation features to maintain the quality of the annotated data.
- Export Options: Compatibility with various data formats is crucial for integrating labeled data into training pipelines.
Benefits of Using Image Segmentation Labeling Tools
The advantages of utilizing an image segmentation labeling tool extend beyond just efficiency. Here are some critical benefits:
1. Enhanced Efficiency
Image segmentation labeling tools automate various aspects of the annotation process, significantly reducing the time needed to label extensive datasets. This automation allows teams to focus on higher-value tasks like model training and optimization, rather than getting bogged down in manual labeling.
2. Improved Accuracy
Tools that incorporate machine learning can offer predictive suggestions and corrections, which help improve the accuracy and consistency of the labeled data. This, in turn, leads to better-performing AI models. With image segmentation, the possibility of human error is greatly minimized, ensuring that every pixel is labeled correctly.
3. Cost-Effective Solutions
By enhancing efficiency and accuracy, image segmentation labeling tools lead to cost savings over time. Reduced time on projects translates to lower labor costs and faster delivery of services and products to market.
4. Scalability
As your business grows, so do your data annotation needs. A robust image segmentation labeling tool can easily scale to handle increased volumes of data, making it a future-proof solution.
Choosing the Right Image Segmentation Labeling Tool
When deciding on an image segmentation labeling tool, it’s essential to evaluate your specific needs and constraints. Here are some crucial steps to guide your choice:
- Assess Your Requirements: Determine the volume of data, types of images, and the level of detail required for your annotation tasks.
- Evaluate Scalability: Check if the tool can adapt to increasing data loads over time.
- Consider Integration: Ensure the tool can integrate seamlessly with your existing data workflow and software.
- Read Reviews and Case Studies: Look for user feedback and performance reports from other businesses in your industry.
Case Study: Keylabs and Image Segmentation Labeling Tool Implementation
At Keylabs.ai, we recognize the transformative potential of image segmentation labeling tools in improving our data annotation processes. Our team recently integrated a cutting-edge tool that enabled us to optimize our annotation workflow significantly. Here’s how we approached the integration:
Step 1: We began by assessing our existing annotation processes, identifying bottlenecks and areas for improvement.
Step 2: After evaluating several tools, we selected one that offered both automated suggestions and manual control, ensuring our annotators could work efficiently.
Step 3: We trained our team on the new tool, emphasizing the importance of accuracy and consistency, considering both semantic and instance segmentation.
Step 4: Post-implementation, we monitored the project’s progress, analyzing the time saved and improvements in annotation accuracy.
This structured approach has not only enhanced our productivity but has also led to a higher quality of labeled data, thereby improving the performance of our AI models.
Future Trends in Image Segmentation and Data Annotation
The field of data annotation and image segmentation is rapidly evolving, with new technologies and methodologies emerging continually. Here are some anticipated trends that could shape the future:
- Increased Use of AI and Automation: As deep learning technologies advance, more sophisticated machine learning models will be developed to automate data annotation processes, reducing the need for human involvement.
- Enhanced Real-Time Annotation: Future tools may allow for real-time data annotation during data collection, increasing workflow efficiency.
- Integration with Augmented Reality (AR) and Virtual Reality (VR): As AR and VR gain traction, image segmentation will become vital for training models in these environments.
Conclusion
In summary, an image segmentation labeling tool is an invaluable asset for any business engaging in data annotation. As we continue to see advancements in technology and increasing demands for high-quality labeled datasets, leveraging such tools becomes essential for maintaining competitiveness in the market. At Keylabs.ai, we are committed to staying at the forefront of these technologies, ensuring that our clients receive the best possible services and solutions in data annotation.
By understanding the importance of accurate image segmentation, choosing the right tools, and embracing the future trends in data annotation, businesses can harness the full potential of AI and machine learning. As the landscape continues to evolve, those who invest in the right data annotation platform will undoubtedly see significant returns in efficiency, accuracy, and overall success.